Anomaly Detection for Industrial Control Systems Through Totally Integrated Automation Portal Project History
Journal of Wireless Mobile Networks, Ubiquitous Computing, and Dependable Applications (JoWUA). Bd. 13. H. 3. Innovative Information Science & Technology Research Group (ISYOU) 2022 S. 4 - 24
Erscheinungsjahr: 2022
ISBN/ISSN: 2093-5382
Publikationstyp: Zeitschriftenaufsatz
Sprache: Englisch
Doi/URN: 10.22667/JOWUA.2022.09.30.004
Geprüft | Bibliothek |
Inhaltszusammenfassung
Attacks on industrial control systems (ICS) have been intensively studied during the last decade. Malicious alternations of ICS can appear in several different ways, e.g., in changed network traffic patterns or in modified data stored on ICS components. While several heuristics and machine learning methods have been proposed to analyze different types of ICS data regarding anomalies, no work is known that uses the data of Totally Integrated Automation (TIA) Portal for anomaly detection. TIA P...Attacks on industrial control systems (ICS) have been intensively studied during the last decade. Malicious alternations of ICS can appear in several different ways, e.g., in changed network traffic patterns or in modified data stored on ICS components. While several heuristics and machine learning methods have been proposed to analyze different types of ICS data regarding anomalies, no work is known that uses the data of Totally Integrated Automation (TIA) Portal for anomaly detection. TIA Portal is a popular software system for organizing the ICS, with which configuration and programming data can be viewed, changed and deleted. By saving the single project datasets historically, old versions of the current system configurations can be restored. This work extends our previous work [1], in which we started to examine real TIA Portal project data of an automotive manufacturer’s production line, covering a period of about three years of historical data, for various features that may indicate anomalies. We therefore proposed heuristics that detect timing- and size-based anomalies in the TIA Portal data. Our initial approach is extended by applying machine learning algorithms on top of our built heuristics to improve our detection results. We have also added more details of the given dataset. Additionally, we investigate a further feature set consisting of the different types and a varying amount of code blocks of our given dataset. Our approach covers both, changes to the data caused by infiltrated attacks as well as malicious changes made by employees who have direct access to the machines. » weiterlesen» einklappen
Klassifikation
DFG Fachgebiet:
Informatik
DDC Sachgruppe:
Informatik
Verknüpfte Personen
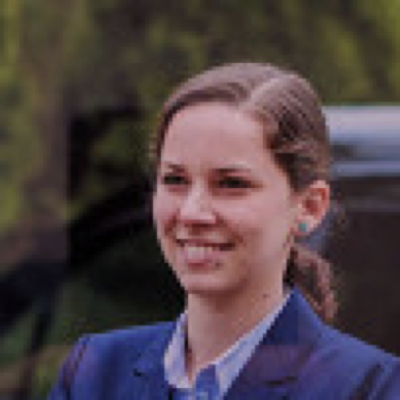
- Laura Hartmann
- Doktorandin
(Zentrum für Technologie und Transfer | ZTT)
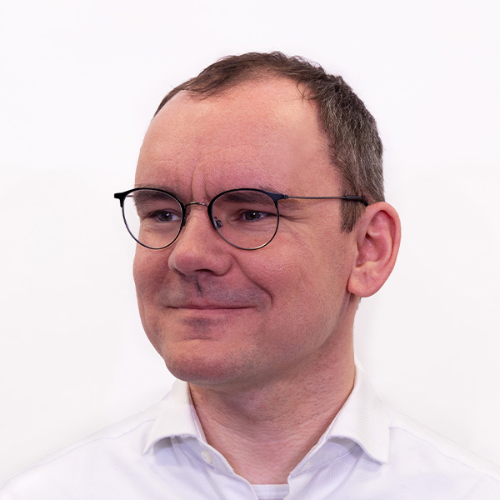
- Steffen Wendzel
- Wissenschaftlicher Leiter
(Zentrum für Technologie und Transfer | ZTT)